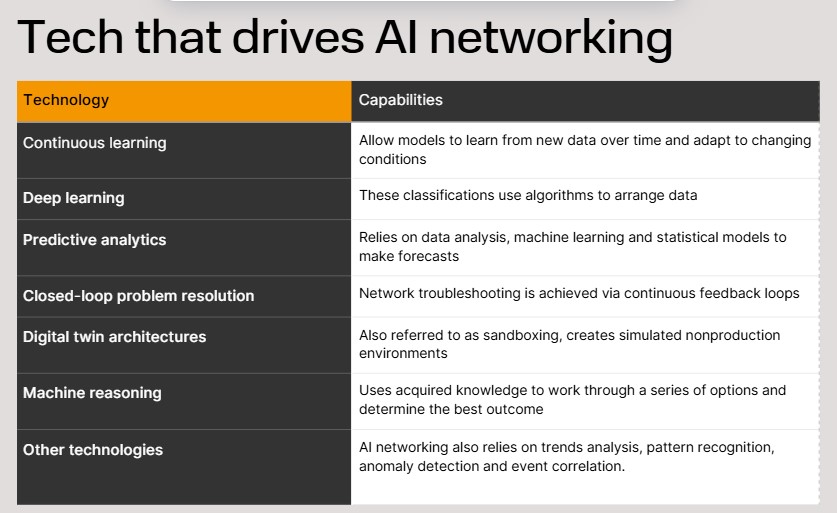
Today’s networks face a multitude of challenges as they become more complex and distributed. Legacy issues around performance, speed, availability and latency still cause headaches, and outages risk financial and reputational damage — just look at the CrowdStrike outage ramifications, which will no doubt continue for years to come.
But AI holds the potential to improve IT networks. The emerging concept of AI networking combines several technologies to automate network operations and help manage networks to improve availability and performance. Among other capabilities, models can configure, monitor, troubleshoot and secure networks and provide incident management and detailed recommendation and response.
So what exactly is AI networking, how does it work and what are its top use cases? Let’s explore this technology that has the promise to transform network operations.
Addressing day 2 operations for reliability, efficiency, performance
AI networking deeply integrates AI into networking infrastructure to automate numerous processes and improve efficiency, adaptability, performance, speed, latency and other critical factors. The term was coined by Gartner in 2023, but the concept has existed in different iterations for some time, with other vendors and analysts referring to it as autonomous networking, intent-based networking, and self-driven or self-healing networking.
[Related: Networking terms and definitions]
There are many phases in a network, from day 0 (initial planning) to day N (end-of-life). AI networking primarily addresses day 2 operations (ongoing maintenance), although going forward it will likely be increasingly applied to day 0 and day 1 (network development and deployment) functions.
For day 2, AI can be used to allocate resources, identify and quickly address (and predict) problems in the network, centralize problem identification, automate recommendation and response, resolve lower-level support issues and reduce trouble ticket false positives through confirm-reject analysis, among other capabilities.
The end goal is to make networks more reliable, efficient and performant. Eventually, networks could also become more autonomous and self-healing (that is, able to address issues without the need for human intervention).
Gartner predicts that by 2027, 90% of enterprises will use AI to automate day 2 operations, up from just 10% in 2023. The firm also says that AI networking can already lower operational management costs by 25% as it improves troubleshooting and networking availability and reduces support calls.
“AI networking promises to deliver improved network availability, performance and operational efficiency,” write Gartner analysts Jonathan Forest, Andrew Lerner and Tim Zimmerman.
IDG
The core components of AI networking
It’s important to note that the use of AI and machine learning (ML) in network management is not new. Artificial intelligence for IT operations (AIOps), for instance, is a common practice that uses automation to improve broader IT operations.
AI networking is specific to the network itself, covering domains including multi-cloud software, wired and wireless LAN, data center switching, SD-WAN and managed network services (MNS). The rapid emergence of generative AI, in particular, has brought AI networking to the fore, as enterprise leaders are rethinking every single aspect of their business, networking included.
AI networking leverages several advanced techniques to automate processes and monitor networks, listed in the table below:
Critical use cases of AI Networking
Let’s dive into some use cases of AI networking. AI-driven networks can do the following:
- Analyze traffic in real time to help ensure the network runs smoothly. This is particularly helpful for enterprises with high traffic that must ensure fast and reliable access and reduce bottlenecks.
- Support capacity planning, resource allocation and help maintain optimal network performance and flow through analysis usage trends to predict future demands. AI can assess network health through performance trends and compare those with industry peers.
- Perform longer-term prediction modeling to determine when network outages or degradation might occur. It can also identify latency problems or congestion and take action to reroute traffic, scale infrastructure or otherwise distribute network resources.
- Optimize IT service management (ITSM) by handling basic troubleshooting (level 1 and level 2 support) such as password resets or simple hardware glitches. It can also help to eliminate false positives and identify higher-level issues that require human intervention.
- Improve threat response, identify and assess security incidents and offer remediation actions. Augmented with zero-trust configuration, AI can identify and classify devices on the network and analyze traffic, logs and user behavior to identify anything suspicious and provide alerts in the event of a cyberattack or breach (or attempted ones).
- Tailor network experiences for different user groups to meet specific needs and improve productivity. This is particularly important in larger environments with diverse groups of users.
- Track internet of things (IoT) endpoints to help with deployment, maintenance and troubleshooting.
- Automate policy by analyzing traffic flows (protocol, port numbers, source and destination) to permit or deny certain interactions between users, devices and apps.
- Improve lifecycle management by assessing devices and verifying that they all have the latest software. It can look for potential configuration vulnerabilities or issues and provide suggestions for upgrades.
Current challenges in AI networking
Of course, no technology — no matter how long it’s been around — is challenge-free. Particularly because it is such a new concept, there are always issues around inflated expectations, overstated capabilities and oversetting expectations. There are also always concerns about cost with any new technology.
No matter how impressive and groundbreaking AI might be, it can be wrong, too. It can make inaccurate recommendations that result in incorrect or overly complex network configurations, outages and other issues. This might be caused by incorrect prompts or insufficient, subpar-quality, inaccurate or non-relevant training data.
New technologies also require new skills (knowing how to prompt, for instance, or understand ensuing outputs), so enterprises have to commit time and resources to upskill or reskill employees. Dovetailing with this is cultural buy-in, as workers may be risk-averse, distrusting of AI, fearful that it may replace their jobs, or simply have no interest in learning new skills.
How to get started with an AI networking strategy
AI networking solutions are offered through a variety of different formats. It can be incorporated into existing AIOps platforms as part of a horizontal approach; provided as a feature within a network vendor’s platform; offered as a stand-alone capability across multiple vendors; or delivered as a managed network-as-a-service (NaaS).
It’s critically important to select the right architecture for your enterprise to ensure the best outcomes. Tools must integrate within existing systems, support Day 0 to Day N use cases and provide the ability to scale with network growth.
Before moving forward with any solution, first gain a strong understanding of your network needs. Assess current network infrastructure, understand challenges and requirements and identify areas where AI might be most beneficial.
Gartner suggests starting small and performing proof of concept (PoC) tests before moving tools into production. In sandboxing environments, act on a model’s recommendations and predictions and test their accuracy, then rely on automation as systems prove themselves (or don’t) and human trust increases.
Identify what type of AI networking system — NaaS, AIOps platforms, vendors, multi-vendors or managed service providers — is best for your business based on existing resources and needs, Gartner advises. Determine whether a vendor’s operational model is MNS, DIY and single or multi-vendor, and require them to provide specific details and breakdowns of their tools, what they can and cannot do, and offer clear timelines from implementation to a few years out.
NaaS company Nile urges enterprise leaders to invest in systems that efficiently collect and process data and are routinely retrained. To help keep up with cyber threats, ensure that solutions adhere to security standards and compliance requirements, the company advises. Also, understand — and be prepared for — the cost of implementation, which can vary from vendor to vendor.
Just as importantly, give IT teams the tools they need to act on AI recommendations and assess how network operations may (fundamentally) change, Gartner emphasizes. Finally, justify adoption by calculating cost savings and benefits around network availability and performance and resource efficiency.
The wrap on AI networking
AI networking has the potential to transform and modernize IT networks. There are many opportunities — and important considerations and challenges, too. As with any technology, it is critical to take a strategic approach, iterate carefully and educate, train and constantly upskill to gain the greatest benefits.
Source:: Network World